In today’s AI-driven and geospatially enabled world, data is an organization’s most valuable asset — yet it is often treated as an afterthought until issues arise. Poor data quality, incomplete metadata, and inconsistent governance can quickly derail even the most sophisticated projects. At Cercana, we believe that data stewardship must be intentional, continuous, strategic, and embedded into every phase of the project lifecycle.
Why Data Stewardship Matters More Than Ever
The rapid adoption of artificial intelligence and machine learning means that models are only as good as the data that train them. In geospatial systems, slight inaccuracies — such as misaligned coordinates or outdated basemaps — can cascade into serious operational errors. Furthermore, organizations are increasingly judged not only on the outcomes they produce but also the quality and governance of the data they maintain, particularly under frameworks such as GDPR and emerging AI regulations (Voigt & von dem Bussche, 2017). Effective data stewardship is now essential for both technical success and regulatory compliance.
Research indicates that up to 80% of AI project failures can be traced back to issues of poor data quality (Gartner, 2021). Organizations that invest early in stewardship practices stand a much greater chance of building reliable, resilient systems.
Common Pitfalls When Stewardship Is Ignored
When stewardship is neglected common problems emerge quickly. Disparate data sources lead to inconsistencies that degrade model performance and decision-making. Metadata is often incomplete, particularly for spatial attributes like projection information or temporal validity, limiting future usability. Without lineage tracking, teams cannot verify the origin or reliability of their data, making validation nearly impossible. Additionally, mismatches in coordinate systems, uncontrolled enrichment, and poorly managed access rights introduces risks that compound over time. Without a defined stewardship process even the most promising initiatives can stagnate or fail outright.
Security Risks Tied to Poor Stewardship
In addition to operational challenges, poor data stewardship also introduces serious security risks. Mismanaged datasets can unintentionally expose sensitive information, particularly when metadata or spatial attributes reveal more than intended. Without proper lineage tracking and access control, organizations are vulnerable to unauthorized data manipulation, leakage, or corruption. Furthermore, compliance with emerging security and privacy standards increasingly depends on maintaining disciplined data governance practices (NIST, 2020). Strong stewardship is not only essential for quality and reliability — it is also critical for protecting organizational and national security interests.
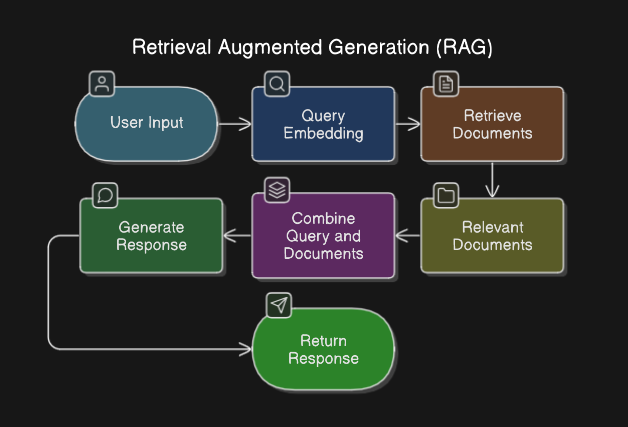
Retrieval-Augmented Generation (RAG) systems, which combine data retrieval with AI driven content generation, are particularly vulnerable to a form of attack known as RAG poisoning. In this scenario, malicious or inaccurate data is intentionally inserted into the knowledge base leading the AI to retrieve and generate harmful or misleading outputs. Without strong data stewardship practices, including strict validation, provenance tracking and controlled ingestion pipelines, organizations may unwittingly expose themselves to these sophisticated new threats.
What Effective Data Stewardship Looks Like
Effective stewardship begins with discovery; cataloging all datasets including third-party and open-source feeds. Standardization of schemas, metadata fields and coordinate systems follows, ensuring consistency across applications. Enrichment activities such as gap-filling, normalization, and validation against authoritative sources elevates the quality of data available for analysis. Governance frameworks define who can create, edit, validate and retire datasets, providing necessary accountability. Finally, continuous monitoring using audits and stewardship KPIs ensures that quality standards are sustained overtime (Redman, 2018).
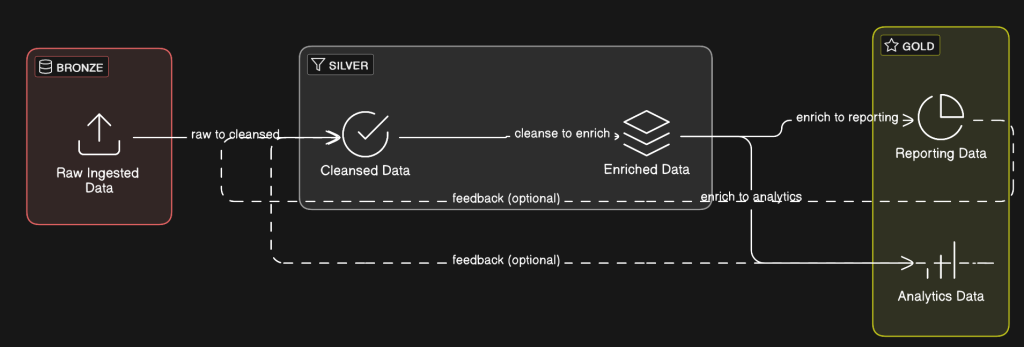
Many modern data platforms implement these stewardship principles using structured frameworks like the Medallion Architecture, which organizes data into Bronze (raw), Silver (cleaned) and Gold (curated) layers. This structured progression enforces discovery, standardization, enrichment and governance practices in a scalable way (Armbrust, Das, Zhu, & Xin, 2021). By applying stewardship systematically across each stage, organizations can build more resilient and trustworthy AI and geospatial systems.
Organizations leveraging open datasets must pay particular attention to validation as not all external sources meet the same quality thresholds required for mission-critical work.
How Cercana Helps Clients Get It Right
At Cercana, data stewardship is foundational not optional. We work with leading technologies such as Apache Airflow for orchestration, dbt for data transformation, Delta Lake for storage reliability, and PostGIS for advanced geospatial data management. We embed stewardship practices at the earliest phases of our projects ensuring strong, reliable data pipelines from day one. Our team brings expertise across metadata cataloging, ETL/ELT pipelines, geospatial validation and stewardship strategy development. Beyond tools and processes, we assist organizations in building a sustainable stewardship culture through team training and change management. We believe that good stewardship is as much about people and processes as it is about technology.
Conclusion
Data stewardship is no longer a back-office concern; it is a mission critical capability that underpins the success of AI, machine learning and geospatial analytics initiatives. Organizations that prioritize stewardship today will be best positioned to lead in an AI-driven, regulation-conscious future. To learn how Cercana can help you strengthen your data stewardship practices, contact us today.
References
Armbrust, M., Das, T., Zhu, X., & Xin, R. (2021). Delta Lake: High-performance ACID table storage over cloud object stores. Proceedings of the VLDB Endowment, 13(12), 3411–3424. https://doi.org/10.14778/3415478.3415560
Gartner. (2021). Gartner predicts 80% of AI projects will remain alchemy, run by wizards whose talents won’t scale. Retrieved from https://www.gartner.com/en/newsroom/press-releases/2021-03-17-gartner-predicts-80–of-ai-projects-will-stagnate
NIST. (2020). Security and Privacy Controls for Information Systems and Organizations (NIST Special Publication 800-53 Rev. 5). National Institute of Standards and Technology. https://doi.org/10.6028/NIST.SP.800-53r5
Redman, T. C. (2018). Data Driven: Profiting from Your Most Important Business Asset. Harvard Business Review Press.
Voigt, P., & von dem Bussche, A. (2017). The EU General Data Protection Regulation (GDPR): A Practical Guide. Springer.